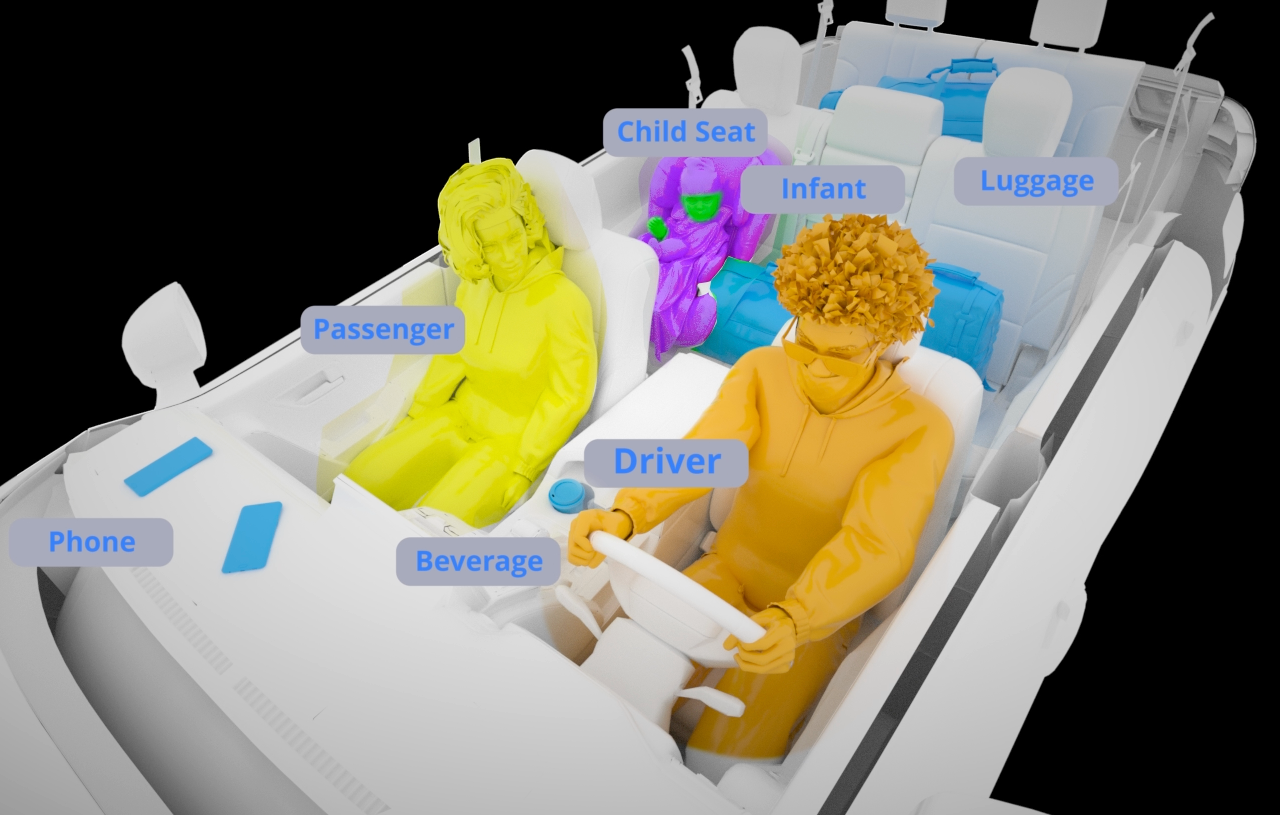
Synthetic data is the key to improving road safety
Human error is the cause of nearly all road traffic incidents, which are now the leading cause of death in the United States for people aged 1-54. In 2020, almost 39,000 fatalities were recorded on the roads in the US alone – the vast majority being attributed to drivers making mistakes. On top of this, over 2 million people were injured in a staggering 5.25 million non-fatal crashes. And that was Covid’s year, with lockdowns and travel restrictions greatly reducing some of the more usual numbers.
Until recently, the vast majority of cars have been severely limited in their ability to improve overall road safety. Mechanical monitoring systems, like seat occupancy and seat belt-fastened sensors, have been around for quite some time, but they’re often blunt in implementation and rarely work in conjunction with other support systems in the car.
With recent advances in hardware and processing power, camera-based monitoring systems powered by Machine Learning are poised to make these shortcomings a thing of the past. This is due to their superior ability to detect a wide range of risky behaviors – from the obvious ones to the almost imperceptible human behaviors.
While most in-cabin safety systems powered by Machine Learning are still evolving, the need for more intelligent tools to make our journeys safer continues to accelerate. This is where the next generations of Driver and Occupant Monitoring Systems (DMS and OMS) come into play, detecting in-cabin hazards that could lead to potentially dangerous situations on the road.
Driver Monitoring Systems (DMS), as the name suggests, focus on the driver, detecting subtle signs of distraction or drowsiness. Incoming guidelines from car safety assessment programme Euro NCAP will require all new cars in Europe to be fitted with a DMS by 2026 at latest. However, with organizations aiming to boost vehicle safety even further, the automotive industry is increasingly moving towards Occupant Monitoring Systems. These go beyond the driver to focus on other things within the cabin such as identifying the number of passengers in the vehicle – regardless of whether they’re sitting in between seats, or standing on the footwell – or the presence of pets or an incorrectly installed child seat. With incoming regulations requiring cameras in every vehicle, it makes sense to leverage them to detect other potential hazards, beyond the driver.
Both DMS and OMS are powered by Machine Learning networks. To perform at their best, they need to be trained on large sets of high-quality training data – in particular, images and video recordings that capture as many different and diverse in-cabin scenarios as possible, like drivers becoming fatigued, using phones, smoking cigarettes, or drinking at the wheel. Using cameras and actors to capture real images of every imaginable scenario would be incredibly costly and nearly impossible. Yet the performance of DMS and OMS systems depends heavily on the quantity and the quality of the data used for training and validating the networks.
Producing this kind of training data synthetically makes it possible to tweak almost any variable and generate new imagery in a matter of seconds. That means we can create a unique scenario, and then produce hundreds of permutations of the same training sequence with different characters featuring slight variations, from age and ethnicity, to clothing, glasses, and face masks. All provided with their metadata counterparts for use during the validation process. Producing such a wide range of training images in real life would take an unfeasibly large amount of time, organization, and money, while configuring these different variations using synthetic data takes mere minutes.
Not only is synthetic data cheaper to produce than real-world imagery, it also cuts out any issues surrounding privacy. And it covers situations that would just be too dangerous or too difficult to replicate. The speed at which synthetic data can be produced also means that new features can be brought to market much faster.
Data quality is absolutely key when it comes to training and validating the Machine Learning networks that power in-cabin DMS and OMS. What’s more, taking a metadata-first approach means that datasets produced are not just highly detailed, but also guaranteed to include exactly what each unique Machine Learning network would need to understand. In practice, this means that training data can include not only the things that humans can see, but also the tiny details that are often filtered out by our senses, such as micro movements of the eye, which could denote the difference between someone falling asleep, or simply looking down. Devant’s platform is capable of generating the most comprehensive and accurate training data in the world for Machine Learning.
The other big advantage is that automotive manufacturers can continue to iterate on new edge cases, updating their in-cabin safety technology with new features, or enabling it to detect new potential dangers – such as the use of e-cigarettes and vapes – without having to recreate complex shoots in real life. This will enable car makers and aviation companies to keep updating and improving their safety systems faster than if they were to train them on data captured by real cameras, involving the time and cost of coordinating a production crew, actors, a fleet of cars, and more.
The move from DMS to OMS will see a natural surge in the requirement for customizable, granular imagery to feed ML networks with the data required. What’s necessary is broad, high-quality data generation at scale without compromising on delivery times.
Machine Learning-based systems will be able to detect more and more subtle details that could aid disaster prevention. This brings the potential for completely new features, such as the ability to detect potentially hazardous heavy objects on the back seat of a car and deploy extra airbags to protect the driver and front-seat passenger.
The use of high-quality training data also opens up the potential to improve and combine the capabilities of existing sensors, and make them work together more effectively. For example, sensors that have been present on cars for some time, such as rear-view parking detectors, could be combined with in-cabin sensors to detect dangers in a more natural and realistic way.
There is plenty of scope for the use of DMS and OMS in-cabin systems trained and validated on datasets with sufficient data coverage to expand beyond the consumer car and commercial aviation markets, into areas such as public transport, maritime, and the fleet vehicle sector. So far, we’ve only scratched the surface of what high-quality synthetic data can do for in-cabin safety.